Question: The AI copilot—I expected something like that before an autonomous car. Can you talk about the timing of that?
Huang: It’s really hard. It’s way harder than an autonomous car. I showed four capabilities. All four capabilities didn’t exist until recently. One singular AI is able to watch where your eyes are looking, where your head is pointing, reading your lips. We’re doing face perception in real time. Something you and I do very easily, very naturally, is really hard for a computer to do. This camera is going to sit inside your car and monitor everything. It’s watching you, watching your passengers.
Question: So it takes more compute power to do that than–
Huang: The full Xavier. I know. It makes sense. These networks are really big. They’re really deep. Gaze tracking is easy to do poorly, but it’s really hard to do well. Lip reading wasn’t even possible until recently. I mentioned the folks at Oxford we worked with, who inspired this work. It’s very hard.
AI Weekly
The must-read newsletter for AI and Big Data industry written by Khari Johnson, Kyle Wiggers, and Seth Colaner.
Included with VentureBeat Insider and VentureBeat VIP memberships.
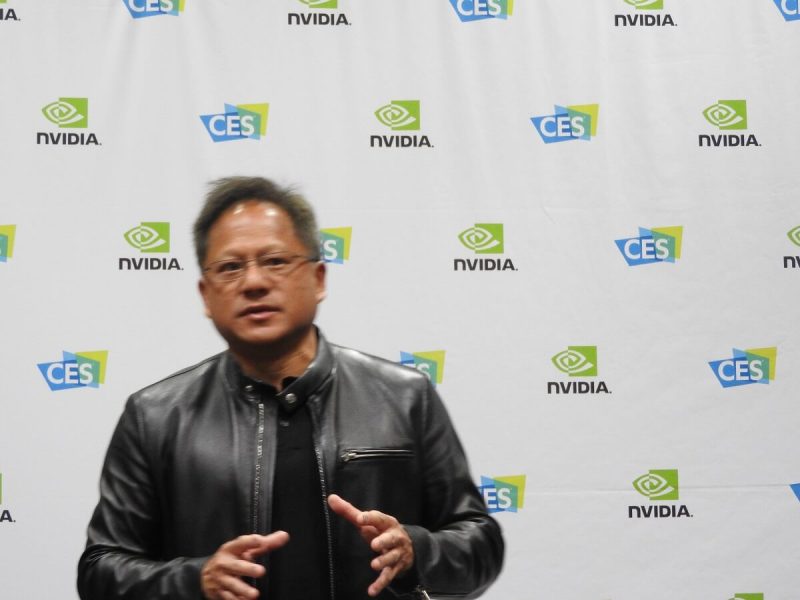
Above: Jen-Hsun Huang, CEO of Nvidia, at CES 2017.
Question: Does it mean that you’ve dropped voice recognition?
Huang: We’ll do both. But sometimes maybe the environment is too noisy. Maybe you have your windows down. Maybe it’s a convertible. We can still read your lips. It makes sense, right? This car is now an AI, and it has to monitor the driver. But it’s also monitoring all of the environment. Where’s the motorcycle? Where’s the bicycle? Are there pedestrians? Is there a kid playing in the street? Did a ball just roll in front of the car? All this stuff is happening in real time. Copiloting, as it turns out, is really hard, even though it doesn’t drive.
Question: Given Intel and Qualcomm’s offerings, this market seems to be getting very crowded now. What do you think is your competitive advantage? What are the defining factors that separate winners from losers in the autonomous car computing market?
Huang: First of all, it’s a hard problem. It’s a visual computing problem and an AI computing problem. Those two things, we’re incredibly good at them. We’ve dedicated a lot of time to mastering this field. It’s the type of work that we selected, because it’s the type of work that we would be good at. We started working on AI cars, self-driving cars, long before it was going to be a successful market. For the first nine years, while I was working on this, it was largely a non-market. Zero market. We chose it, though, because the work is important. The work is hard, but it’s something I believed we could be good at.
Now it’s going to be a very large market. There’s a lot of entrants, as you say. But the fact of the matter is, right now there are very few solutions in the marketplace. Drive PX is not designed to be a demo. It’s designed for production. We’re the only production platform in the world today running on Model S. It’s just started shipping this last month. My sense is that we’re going to be ahead of the world in shipping production for real level four self-driving cars, probably by about three years.
Question: Talking about Nvidia transforming into an AI company is interesting. Does that mean you leave some work in gaming and graphics behind, when you’re prioritizing and doing resource allocation? Are you putting a lot more of your R&D and engineering, people going to work on AI, as opposed to your traditional business?
Huang: We have a lot of people working on AI. That’s surely the case. When I think about the work that we do, it all has one thing in common. Number one, it’s all based on GPU computing. We select work that we do not based on whether we think the market is going to be big or not. You guys have known me for a long time. We select the work we do based on the three things I said to this gentleman just now. Is the work important to do? Is it hard to do? Is it work we’re uniquely good at doing? We select work to do that is consistent with our core competencies, what we’re supposed to focus on.
Almost everything we do is based on GPU computing. We work on four different areas, as you guys know: gaming, virtual reality, AI data centers, and self-driving cars. We only really do those four things. We don’t do anything else. It just turns out that these four things happen to be really cool. They’re really impactful. It’s taken a long time for it to become, if you will, “real.” The reason for that is because it’s hard. But they’re all based on one fundamental thing, GPU computing.
They share a couple of capabilities. One is related to visual and one is related to intelligence, artificial intelligence. Maybe someday somebody will discover that imagination, which is computer graphics, and intelligence, which is AI, deep learning—maybe they’re cousins in some way. The computation of these two problems is highly related. Our ability to imagine and our ability to solve problems could be very similar. I don’t have a philosophical linkage between the two, but the two problems are very similar.
Question: You chose to invest in this market several years ago, and now it happens to be something very important. Did you foresee that shift happening?
Huang: We always work on things that are important. The first question is, “Is this an important problem?” Autonomous vehicles is an important problem. Robotics is an important problem, and a very hard problem. It’s a problem that a company like Nvidia would be very good at solving, because the solution involves visual computing and artificial intelligence. It made sense for us to work on it, even if it took a long time.
The way to think about that is, yes, we absolutely foresaw it coming. That’s why we believed it was important. But it took quite a long time. 10 years is a fairly long time to work on something. But if you enjoy working on something, 10 years comes and goes.
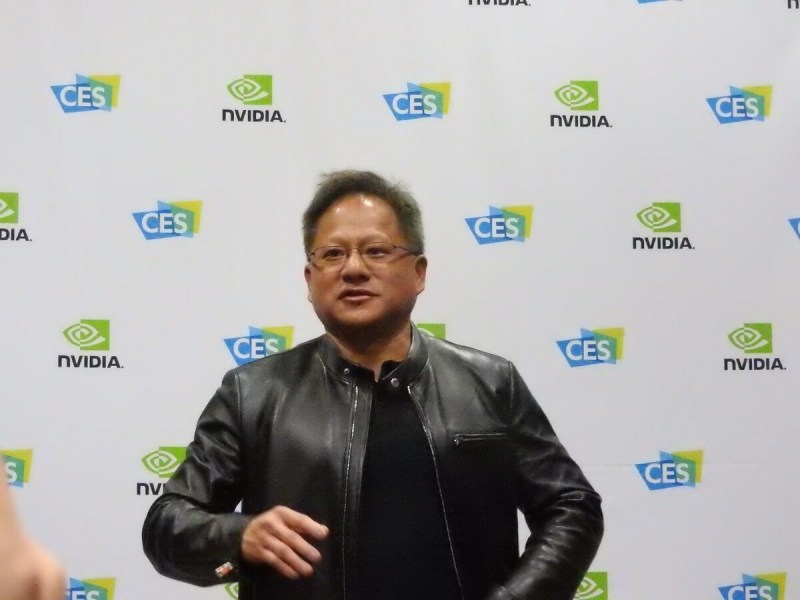
Above: Jen-Hsun Huang, CEO of Nvidia, at CES 2017.
Question: What do you think about reports of accidents in tests of self-driving cars? The Tesla incident was the most prominent.
Huang: It’s really unfortunate. There’s no question that the technology has much to advance. That’s why we’re dedicating so much R&D to it. The problem of self-driving cars is an AI problem, and a really hard one. I just don’t think that the work is done yet. That’s why we’re working so hard at it. It’s obviously a very important problem to solve.
Question: Related to that, one of the challenges that the market is facing is that you have a bunch of companies, yourself included, touting autonomous driving as here and now, yet also saying that it’s not here and now. It gets confusing to reconcile when it’s really here – not one or two cars, or even 40 cars, but millions of cars. Tesla is confusing things even more as to how they define what they’re doing. From your position, how should people think about that? How do we reconcile the present versus the future, what we can do and what we can’t?
Huang: First of all, all of you can control this situation quite well. Just don’t write about it. [laughter] Obviously, the reason why we talk about it, the reason why people are interested, is because transportation is such a big deal. It’s the fabric of society. The internet moves information, but transportation moves things. We need things to live. It’s obviously very interesting. Of course, automobiles also connect with a romantic side of us. We love cars. It’s fun to write about.
Now, I think you have a serious question in there related to how we know how far along this is. I actually don’t feel that most people are confused about the capabilities of their car. Just because they’ve read about this in the news yesterday, they don’t go home and say, “Car, drive.” They know that their cars don’t drive themselves. I drive a Model S. I can tell you that it helps me every single day. It improves my safety.
Question: I guess the question is assisted driving versus autonomous. It seems like everyone is focused on autonomous when assisted is more practical and more useful.
Huang: Maybe what you’re saying is what I’m saying as well, which is—I believe a car, an autonomous vehicle, the first thing it’s going to do is plan a route. This car already has a computer inside, connected to the internet. You say, “I want to go here,” and it’ll plan its route, just like a plane does, just like we all do. Out of that route that it’s planned, parts of it, or all of it, it might be able to do that autonomously. If it can do that confidently, then it’ll do it and do it well.
If parts of that route can’t be done autonomously, it’ll tell you. There are lots of different ways to tell you. We’re saying that even when it’s not driving for you, it should be looking out for you. As a result, this AI car concept is a larger concept than autonomous vehicles. That’s why I didn’t say “autonomous vehicles.” I call it an AI car. I believe this AI car will have two basic functionalities. One, driving for you. Two, looking out for you. That idea, I believe, we can put on the road tomorrow morning and it’ll be a betterment for society. But we do have to finish the technology.
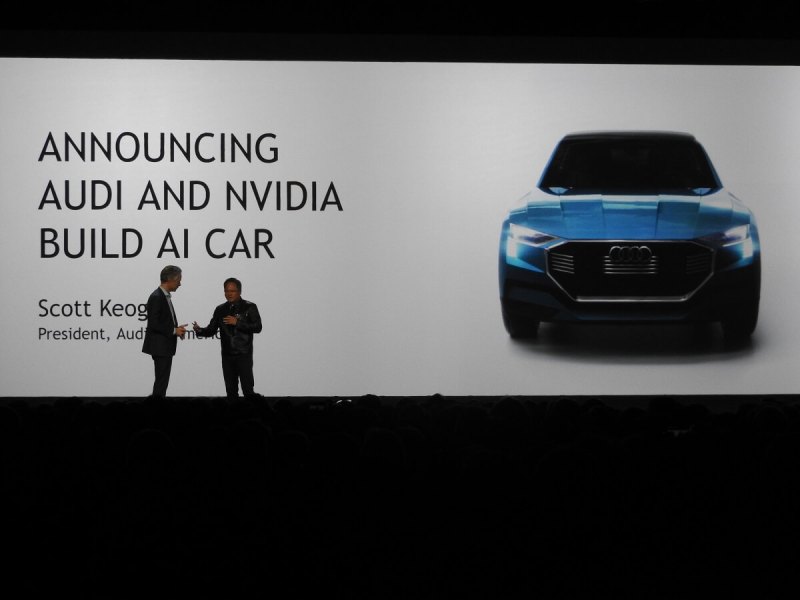
Above: Nvidia has partnered with Audi on AI cars.
Question: You’ve announced a broad partnership in the auto industry. What kind of roles does Nvidia want to play? Just as a hardware supplier, or do you have ambitions to play a bigger part in the ecosystem?
Huang: We’re just trying to solve the problem. Our plan is not nearly as grand as what you may be thinking. We believe that there are a lot of cars in the world, a lot of car makers, a lot of car services. There are trucks, shuttles, vans, buses, automobiles of all different types. There is no one-size-fits-all solution for all of those, because they all have different problems and capabilities.
In the case of a shuttle, it’s geo-fenced. The flexibility of the service doesn’t have to be infinite. You can have a lot more mapping data. In the case of an individually owned car, that car has to go anywhere. You should be able to drive your Mercedes to downtown Bangalore as easily as Mountain View or Shanghai. The capabilities of that car have to be different, and so are its limitations, because the challenges are different.
I would say that, number one, there’s no one solution for everything. However, the computing platform for AI can be consistent. Just as every computer is different, the computing platform underneath – the processor, the operating system, the AIs – can be very similar. Our strategy is to create the computing platform. We call it the Nvidia AI Car Platform. This platform would be used by tier ones when they work with OEMs. It’ll be used by OEMs. It’ll be used by car companies, shuttle companies, so on and so forth.
Question: You showed us, yesterday, the Shield and how it’s connected to the Google Assistant. Why do you need the Google Assistant when you could do your own thing?
Huang: It turns out that Google Assistant is quite an endeavor. There are two pieces of Google Assistant, or let me say three pieces, that are quite hard to do. One of them is speech recognition and synthesis — automatic speech recognition and text-to-speech. On top of that is the layer called natural language understanding. That’s what I said versus what I meant. If I just said, “Open it,” I could have been talking about opening anything. But what I meant was likely related to what I was talking about just previously. The natural language understanding part of AI is really complicated. That’s what Google Assistant is doing. The back end of that is a search engine. Google, as you know, is quite good at search. It’s not an inconsequential amount of capability.
When you’re using Google Assistant, you get used to the capability of that assistant. Once we learn how to use a particular assistant, that assistant has capabilities, strengths and weaknesses, and personality. Over time it’s easy for people to use that capability instead of learning four or five different assistants. I get used to working with the people I work with based on our common understanding of each other. Your assistant’s going to be the same way.
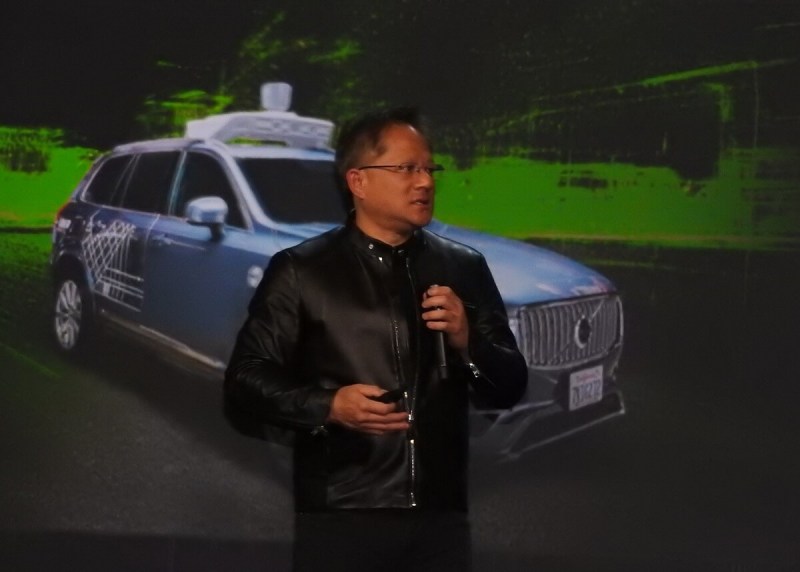
Above: Jen-Hsun Huang, CEO of Nvidia, at CES 2017.
Question: You just mentioned that everything will be an AI problem, and that AI problems are very hard to solve. The biggest companies in the industry are all looking closely at this market. What about smaller companies? How can they keep up and contribute to the market in the future?
Huang: This is a great time for startups. We’re working with 1,500 startups today. Never before in the history of our company have we worked with so many startups.
It’s not completely accurate to say that every problem is an AI problem. It turns out that many tough problems we’ve wanted to solve for a long time are AI problems. We’ve not been able to solve them because of the perception part, the world observation part, the pattern recognition part of that problem is so hard, that we couldn’t solve that part until deep learning came along. Recognizing the information. What am I seeing right now? What’s happening right now? That piece of information is easy for a person, but it’s hard for a computer.
Finally we’ve been able to solve that problem with deep learning. Once that happens, the output is metadata. It’s computer data. Now that computer data exists and we know exactly how to use it. We know how to apply a computer to it. What we’re really seeing is that AI is solving some problems that we’ve never been able to solve before.
With respect to startups, the thing you’re starting to see is that these AI platforms are being put into the cloud. These perception layers–once the AI is trained, it’s an API. It’s a voice recognition API or an image recognition API or a voice synthesis API. These APIs are sitting in the cloud. We can connect them to our own applications and write new applications. Startups can now use all of these cloud services. It could be Watson. It could be Microsoft Cognitive Services.
Question: If I’m a startup and my core value is the data that I own, though—if I give away that data, how do you deal with that challenge?
Huang: I know that people say data is the new oil, something like that? It turns out that we all have our own life experiences. That’s what matters. It’s not true that all of these cloud services have all the data in the world. Nvidia designs chips. We have a lot of data about our chips. It’s inside our company. There’s somebody who’s a fisherman, and they own a lot of data about the temperature of the streams where they live. They own a lot of data about that area’s microclimate. That data doesn’t belong to Amazon. Maybe you have a vineyard in France with its terroir and its own special microclimate. That data only exists right there. It’s not available through Google. It’s your data.
That data can be put to good use, finally. The way to think about it is, it’s not true that everybody’s data is going to belong to these cloud services. It’s just not true. We all have our own data. What you’re going to see is, because of AI, these micro-businesses are going to surge. Maybe it’s a brewery. We see people brewing beer with AI now. They have a lot of data about how they brew beer that’s not available on Amazon. It doesn’t belong to Google. It belongs to them. But they can use that information with an AI engine to discover some new insight.
This is a good time for startups. It’s not the opposite.