On Pinterest.com, I find myself being summoned from various directions, as if I’ve just stepped into a party with my closest friends. Many pins I see are interesting to me — it’s a pleasant feeling.
There’s a mid century modern light brown leather couch. A house with a window lined with dark brown wooden shutters. A shelf made from the back of an iMac. A media console on wheels with iron legs and wooden slats. Cinnamon rolls.
Behind each of these recommendations, there’s a reason. Each one is related to something I’ve previously pinned to my Pinterest boards, or something I’ve viewed before, or something I’ve searched for. And, in the case of the cinnamon rolls — it’s because someone I follow pinned it.
None of this is a coincidence. Pinterest’s engineers have been refining the app’s recommendation systems for years. People in the U.S., like me, are used to this sort of personalization. But now Pinterest has more than 150 million monthly active users, and most of the people joining are outside the U.S. So to continue the growth — usage is up about 50 percent year over year, as last year there were 100 million monthly active users — Pinterest has taken new approaches, including employing artificial intelligence, a faster system for ranking, and localization of content.
AI Weekly
The must-read newsletter for AI and Big Data industry written by Khari Johnson, Kyle Wiggers, and Seth Colaner.
Included with VentureBeat Insider and VentureBeat VIP memberships.
Of course, other up-and-coming companies, like Airbnb and Spotify, are also personalizing and localizing their content to accumulate more users and keep them around. Pinterest stands out thanks to its large, image-heavy data collection that users themselves organize. In the past two years, Pinterest has taken steps to do smarter things with all the images, and it looks like that’s paying off.
“With a lot of the focus that we’ve had going international, you can imagine why visual signals would be really valuable there,” Mohammad Shahangian, Pinterest’s lead data science engineer, told VentureBeat in an interview at Pinterest’s San Francisco headquarters.
Deep learning
Machine learning is at work across all four key parts of Pinterest: home feed, search, related pins, and visual search. Today happens to be the one-year anniversary of the launch of the fourth of those.
The visual search system depends on a hot type of artificial intelligence called deep learning, which involves training artificial neural networks on lots of data — such as, you guessed it, photos in pins! — and then getting the neural networks to make inferences about new data. Apple, Facebook, Google, Microsoft, and other companies are using this approach more and more, now that more data and economical computing power is available than ever before.
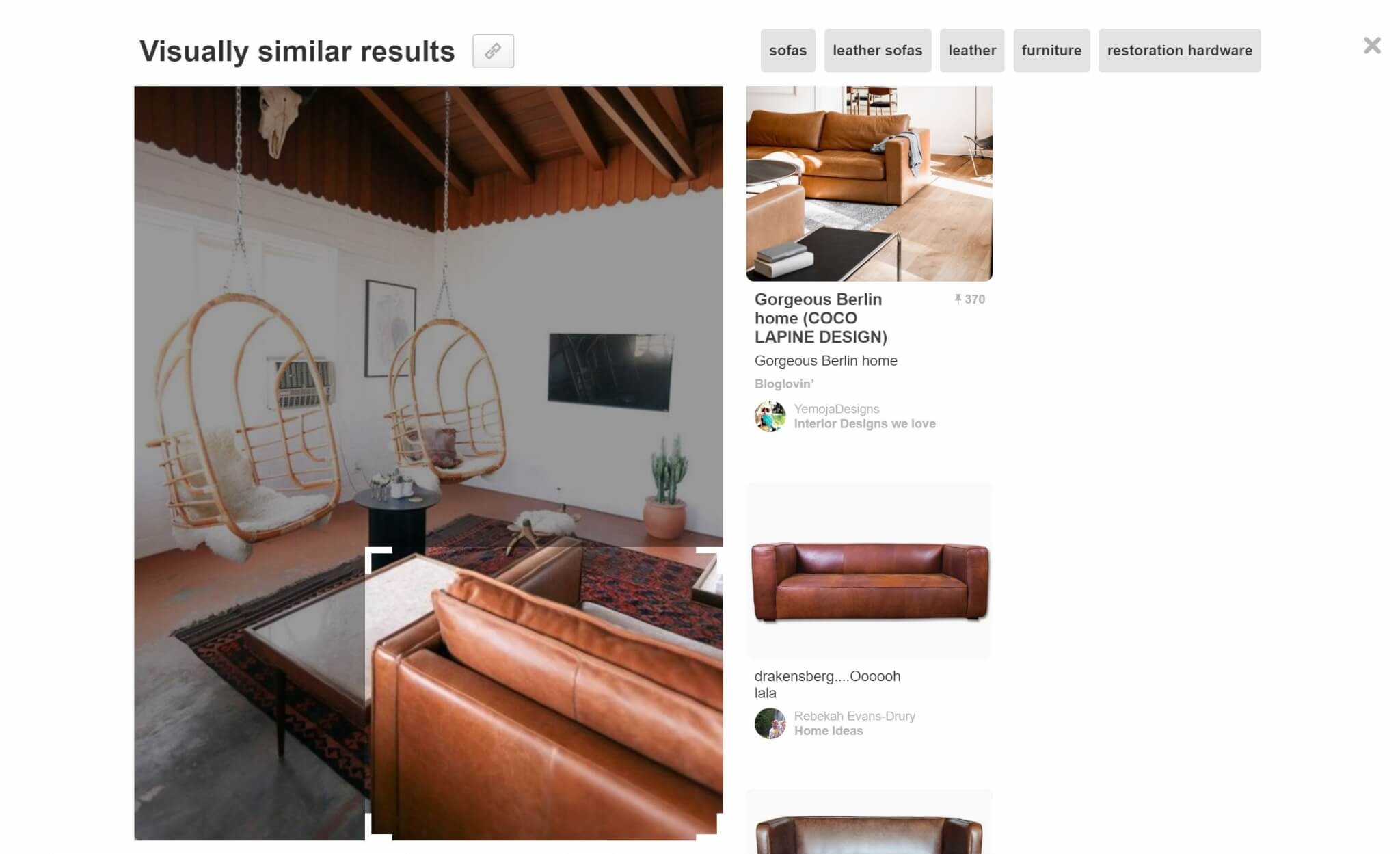
Above: Visually similar search results.
The way Pinterest uses this technology in visual search is certainly compelling. You just click or tap the magnifying glass in the top corner of a pin, and it will let you adjust the size and location of a rectangle superimposed over the image. The software then finds pins that are visually similar to whatever is in the rectangle. Additionally, in some cases you can tap any of the dots that appear on top of objects in a pin to bring up other pins that contain similar objects.
But Pinterest has also begun using deep learning to optimize other parts of its app — like determining related pins.
Pinterest often already knows a lot about something that you’ve just pinned, partly because it knows that some other people have pinned it right alongside one or more other pins. That’s called “co-occurrence,” and it’s a powerful signal that pins are related. But sometimes — say, in another country where many people speak a less-popular language — a user pins something that Pinterest has never seen even once before, and Pinterest can’t do much with the text associated with the pin. That’s where deep learning can make a big contribution. Similarities in the content of the pin can give Pinterest a clue as to what it might be.
And from there, Pinterest is “off to the races in terms of what we can do for your recommendation,” Shahangian said.
But. But! Deep learning is not the primary driver of recommendation systems at Pinterest, even though you might think that image data must be king.
“At the end of the day, you’d always prefer [to ask] ‘What have users curated?’ ‘What have the users done?’ [and use that] as the ultimate signal… as these two things are the most relevant,” said Dmitry Kislyuk, Pinterest’s lead visual search engineer. “That said, there is tremendous opportunity for content, especially content that we know less about.”
Ranking
In May 2015, Pinterest introduced a ranking system to change the order of pins based on how much Pinterest predicted people would engage with pins.
That was big — it led to 30 percent more repins, said Stephanie Rogers, a related pins engineer at the company.
But in January of this year, Pinterest went even further. The company stopped using a batch-processed machine learning system to re-rank content based on what users do, a ranking process that could be done, say, overnight. Instead the company started doing real-time re-ranking, which takes just seconds — fast enough that Pinterest can refine the order of related pins as the person selects a pin to look at more closely.
And Pinterest now takes into consideration your recent activity, like your latest search and the newest pins you’ve saved, when it shows related pins.
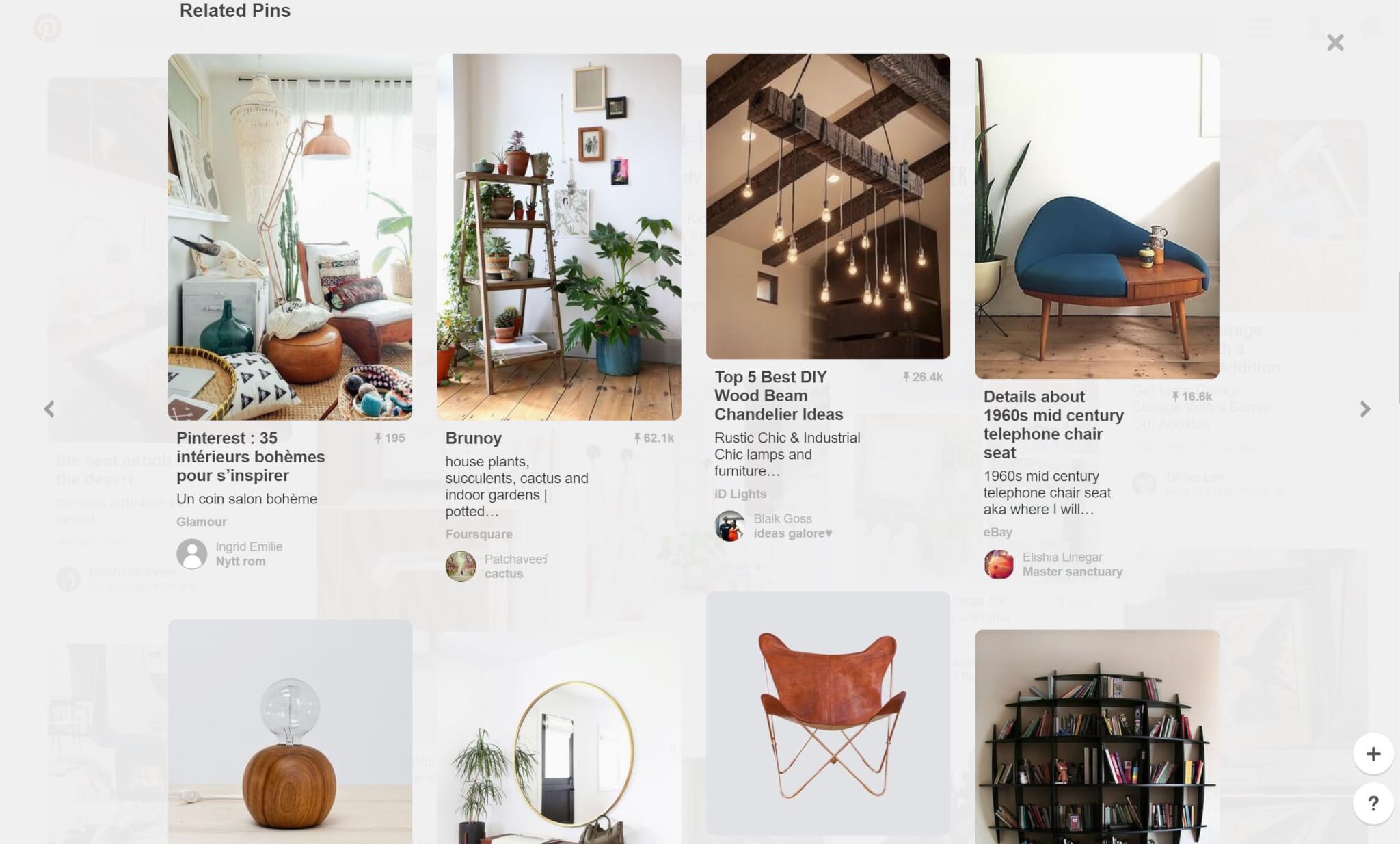
Above: Related pins that have been ranked based on my predicted engagement with theme.
“An example of that might be you were looking up a celebrity, and then you see a celebrity with a certain outfit on, and you click on that pin,” Rogers said. “Related pins should be about that celebrity and not about the outfit [they] were wearing.”
Just adding recent activity into the mix increased the number of pins people save by 4 percent, Rogers said.
Localization
Pinterest has used several tactics to gain traction in foreign countries in the past year.
“Everything is long tail in these emerging countries,” Shahangian said. So deep learning certainly has a role to play. Fast ranking also matters, because the first few things people see can have a big impact on future engagement. There’s more to it, though.
Employees have figured out that some kinds of content — like photography and hair and beauty — do well in many countries, while others don’t. Wedding-related pins from the U.S., for instance, don’t usually play well in other countries.
Pinterest has built machine learning models that focus on just one single part of a category in one country. As certain categories catch on, Pinterest identifies early adopters. Pinterest also looks out for “tastemakers” in certain countries who “time after time bring pins that go on to be successful,” Shahangian said.
And providing content in people’s native language is crucial.

Above: Pinterest as it appears in Germany when a user has selected German as their language.
“Through a variety of techniques, we basically increased local impression share from 8 percent to 54 percent,” Rogers said. In other words, more than half of the content people see is in the language they’ve selected.
It seems that users appreciate that, because in some cases this has led to increased engagement, a spokesperson told VentureBeat in an email.
What comes next
As you might expect, Pinterest has a few ideas about what to do next.
For one thing, Pinterest can start re-ranking visual search results, just like it does for related pins.
It could also start applying deep learning to natural language processing for making certain kinds of recommendations. Pinterest’s ads team has already experimented with that, Kislyuk said.
And it could do more to promote visual search to its users. For example, Pinterest could start talking up its ability to recognize an object given just an image.
That’s possible now, but it’s not obvious — even though it’s impressive and already helping people.
A few weeks ago, Rogers saw a mosque on TV and took a photo of it. She added it to Pinterest. After running a visual search, she was able to find a pin of the same building, and the pin’s description indicated that it was the Vakil Mosque, which is located in Iran. “OK, now I know where this is,” she recalled thinking at the time. “This is awesome.”
Pintastic stats
- 200 million visual searches per month
- 150 million monthly active users, up 50 percent year over year
- 75 billion pins
- 20 million pin recommendation candidates ranked per second
- 10 billion pins being recommended every day, double the number from last year
- 30,000 queries per second for related pins feed
- 250 percent increase in number of localized pins (based on country and language) in the home feed
- 75 percent of sign ups are outside the U.S.
- 40 percent of people signing up are men, and the number is up 70 percent year over year
- 30 percent of engagement comes from related pins
- 20 percent increase in new users outside the U.S. because of changing “Pin it” to “Save”
- 12 percent increase in engagement because of recent home feed algorithm updates
- 10 percent increase in weekly active users due to launch of personalized topics for each user
- 10-15 percent increase in activations after the user experience was redesigned around country-specific topics
- 4 percent increase in number of pins saved and 5 percent increase in localized related pins due to initial attempt to rank related pins based on most recent pins users have saved or searched
- 6 times more likely that someone will save a pin since the launch of object detection in pins; hundreds of millions of objects have now been detected
- 5 times more personalized pins for users outside the U.S.
- 3 times more likely than last year for users outside the U.S. to see localized content in search and related pins
- 3 times faster than the app used to be following overhaul
- 2 times as many users than last year who are running visual searches
VentureBeat's mission is to be a digital town square for technical decision-makers to gain knowledge about transformative enterprise technology and transact. Learn More